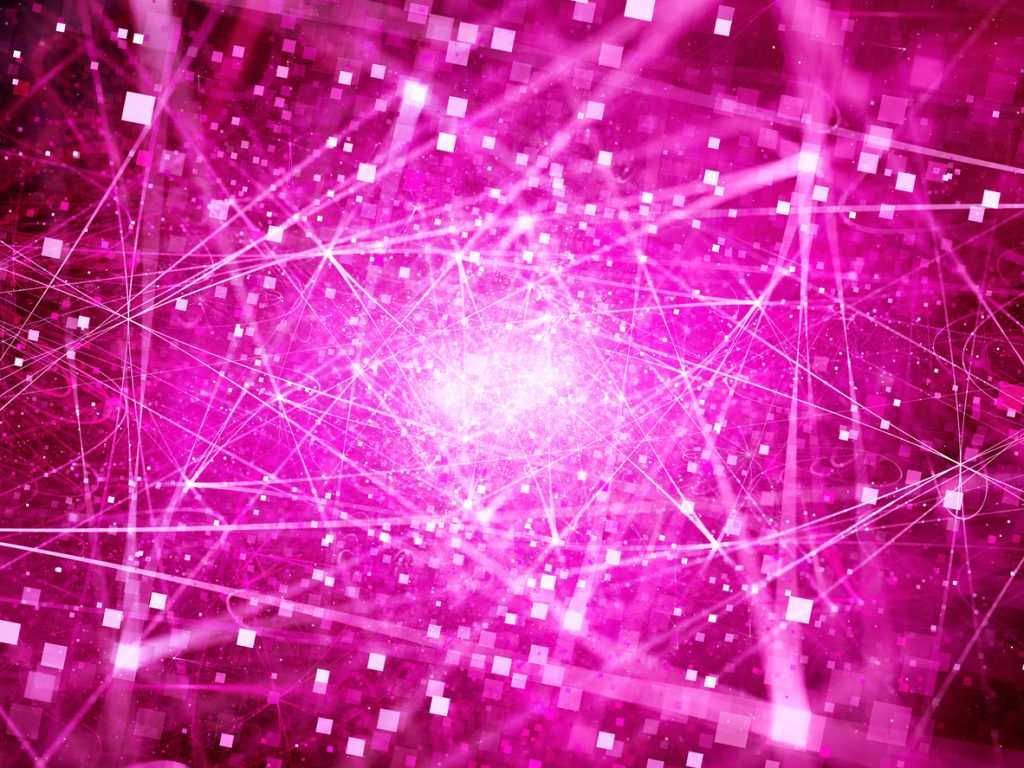
Let’s be frank, shall we?
I have absolutely zero interest in writing about bias.
This subject is more loaded than a Bucky Bee’s one-pound baked potato or an M134 Minigun, if you prefer.
We could all write honestly about bias – honestly because our experiences are ours, unique to us. Everyone has the power to tell their honest-to-goodness truth if they so choose.
To write correctly, though? You need to see the world as it is, not as you are. Sadly, that’s not quite as easy as it may seem on TikTok.
There are many articles about artificial intelligence on this site. More than any other topic, in fact. I would be remiss, not to mention bias. If you’re doing Marketing AI projects involving data, you will encounter it.
So, for this article, I will stick to what I know and write about the types of bias you might see when doing Marketing AI projects, along with a few tips I’ve learned along the way.
TYPES OF AI BIAS YOU MAY HEAR/SEE FREQUENTLY IN MARKETING AI PROJECTS
You probably already know or have encountered several kinds of bias in your life – whether it be about your race, gender, age, physical stature, income level, job title, etc.
When you’re working on Marketing Artificial Intelligence projects, you’re sure to encounter other types of bias. Things like:
Algorithmic Bias – occurs when algorithms produce systematically prejudiced errors that create unfair outcomes. It’s one of the most common forms of AI bias. This is the bias that gets the most headlines.
Anchoring Bias – occurs when systems rely too heavily on pre-existing/historical information or the first information they encounter. This is very prevalent, especially in direct marketing and eCommerce.
Bandwagon Bias – occurs when people adopt a specific behavior or attitude simply because everyone else is doing it. From an AI project perspective, this comes up when using models that include lots of social media data. Bandwagon data can be very disruptive – like a massive blizzard in a climate that’s 70-100 year-round. The data often comes in quickly and disappears even faster, so if you’re not careful, your models can be impacted for a long time.
Blind Spot Bias – occurs when unintended consequences result from oversights in the data, models, training, and workflow.
Confirmation Bias – occurs when one selects only the data that supports/confirms what they already know rather than something that may lead/prove otherwise. In Marketing AI projects, you see this a lot when folks set up their models.
Creator Bias – occurs when the developers/creators of the algorithms use biased rules or data to build them. Sometimes creator biases are intentional (the biases are baked in on purpose), and others are not.
Data Bias – occurs when you use data. This sounds trite, and the reality is that almost all data is biased, starting with how it was acquired, what, where, and when you chose to collect it, and how you use it. The key to minimizing data bias is to know everything about your data, from the first capture to measurement and analysis. This is more doable than it sounds.
Exclusion Bias – occurs when data is inadvertently/inappropriately removed from the data set.
Experimenter Bias – occurs when only some/certain data is recorded, and other data is skipped or left out.
Hidden Bias – occurs when you have unconscious beliefs about different social groups. In the AI world, the term often comes up in a more generic context – meaning something that either The Machine doesn’t see or that the humans don’t see. Ironically, the hidden “something” can be very obvious.
Historical Bias – occurs when the data used to train the AI model/system no longer reflects the current situation. Marketers also use this term when the historical data is so entrenched in the model that the model doesn’t grow/evolve as they want it to or think it should.
In-Group/Out-Group Bias – occurs when we give priority to people who are like us. This is a frequently occurring human bias. In Marketing AI, it tends to impact AI models that involve two or more departments that have conflicting goals.
Measurement Bias – occurs when you don’t correctly/accurately measure/record the data that’s been used. This can be intentional or unintentional.
Outlier Bias – occurs when extreme outliers bias the results. In Marketing, you often see this in dynamic pricing projects, B2B projects involving sales/outbound telemarketing, hybrid B2B and B2C models, and projects involving huge data sets. (The larger the dataset, the harder it can be to find the outliers, but that doesn’t necessarily reduce the impact they may have.)
Sampling Bias – occurs when some data is oversampled, and others are undersampled. Experts say that sampling should be completely random or designed to match the population you want to model. This sounds great in theory, but you may want/need to do something different in practice. The key here is to know what you did and why you did it and then watch how it impacted your models in case you need to disrupt them.
Segmentation Bias – occurs when we slice and dice our file into groups that are too big, too small, or have no meaning/context and then don’t accommodate/prioritize them correctly in our models.
Selection Bias – occurs when we don’t correctly randomize the individuals, groups, or data that we are analyzing. Selection bias becomes an issue when the data does not reflect the population we’re interested in looking at. Marketers tend to obsess over selection bias when using too little or too much data or when using a lot of third-party data.
Societal Bias – occurs when AI reinforces societal biases, intolerance, and discrimination.
User Interaction Bias – occurs when humans intentionally try to influence/bias the results. You’ll often see this as a marketer in projects like internal text search, building merchandising hierarchies, and hyperpersonalization projects.
12 TIPS FOR MARKETERS WHEN IT COMES TO BIAS (aka THINGS I’VE LEARNED THE HARD WAY)*
Depending on what you’re doing and what kind of company you’re working in/with, effectively dealing with bias can be like playing a game of Frogger, except you’re not a speedy frog; you’re a retired turtle who is in lousy shape. My tips come from having recently experienced some big splats. This loosely translates to my tips on bias are, well, biased, but here they are anyway…
Establish precautions to prevent bias at every step of the journey. Marketers often do their bias checks at the end, along with things like accessibility and security. Because bias can easily sneak in anywhere, it’s better to look at bias throughout your project journey – whether you’re planning, building, training, testing, executing, or monitoring. This may seem like extra work, but it ends up being about the same; it’s just front-loaded instead of all at the end.
Be honest about your data. So much of our marketing data is biased. Not because we set out to make it that way but because all our brands and products attract different people. Our target customers are different. Their demographics, psychographics, neurographics, etc., are unique. The key here is to identify what your data is and isn’t so you can adequately accommodate it. What are your data limitations? How are you manipulating the data? What are your analysis and aggregation risks? Where do you have human involvement, and how does that impact you? Remember, AI processes and analyzes oodles of current and historical data in real time and at scale. At least initially, the foundation you start with is often heavily weighted with your old data. If you marketed a specific way or sold to a particular group of people but not to others, then notate it somewhere. Then keep revisiting it as time progresses to decide whether you need to disrupt.
This may sound like a throwaway bullet, but it’s one of the most important. We get so twisted up in our knickers about not being sexist, ageist, racist, etc., that we forget to do realistic checks about how we got to where we are. If you’re a company that exclusively sells outdoor succulents, you’re not likely to have a bunch of North Easterners on your list. Why? Are you discriminatory against all Yankees, or do we just not have the climate to grow 8’ cacti year-round? And yes, I’m aware I will get pinged for this comment and its inherent oversimplification, but alas… This is one of the biggest problems I see in AI right now. Marketers preparing for tsunamis when they’re in land-locked regions.
Speaking of your data… Proper training data is critical. Bias creeps its way into our systems/algorithms in all sorts of ways, but training data is all too often the biggest culprit. Flawed data sampling is another widespread issue. When something is important to us, we often overrepresent it in our training data. Sometimes this is intentional, but usually, it’s not. Sometimes we just don’t have (or use) enough data to train the model(s) correctly. Other times, we’re in a time/cash crunch and cut corners with the training. Often, we don’t know how much representation is enough, or we don’t use enough clear success examples. Bottom line? To have successful AI projects, you must ensure that you have enough training data and that things are adequately represented. “Things” can be people, products, behaviors, etc., depending on what you’re doing.
Document it all, especially when first starting and making disruptions. (Unless your lawyer advises against it, then you do you.) Models grow over time, but what happens at the beginning can substantially impact how things evolve. You don’t need to go into War and Peace length detail, but you should outline any apparent strengths and weaknesses of the data/models you see and any known biases you may have. For example, if you’re building a model to rank leads based on their propensity to buy, and you are using only online data, but most of your conversion happens offline (with sales reps, for example), you’re starting your model on the wrong foot. That doesn’t mean you can’t make it work, just that its base is skewed and likely to get even more twisted. You’ll also want to track how your data was selected, where it’s from and/or enhanced, and how/when it goes through the hygiene process. Update this list as you change things. I’ve found it’s also helpful if you add observations at regular intervals (quarterly, for example.)
Develop a diversified Marketing AI team. This is the recommendation I get the most pushback on, not because people don’t want to do it (they do!) but because it can be challenging. Do it anyway. We can do hard things. How many ethnicities, age groups, religions, income levels, etc., do you need to represent in your project? How much is that going to cost? In my experience, it depends on the project and what you’re using the AI to do. For most projects, it usually turns out to be way more affordable than you think. For the record, you don’t need all the people in-house. You can use outside resources and/or a third-party validator that reviews your models for possible bias.
People often ask: “will I need a diversified team for every Marketing AI project I do?” The answer is no. The takeaway is critically evaluating if your project will benefit from diversity. I get a lot of missile-mails after I say this in speeches, so let me be clear, not all Marketing AI projects need a “team” to begin with. Additionally, many projects don’t use customer data at all. Do I believe in diversified teams? Absolutely. Do I think you should delay getting involved in AI because you’ve read too many clickbait-y headlines about AI? Hard no.
Determine where Governance sits before you start implementing AI. Don’t wait till you have a problem to figure out who is responsible for setting your rules/standards. Identify your guidelines, policies, and practices upfront so that if a problem arises, you can deal with it quickly and easily. This is a good thing to do, even if you’re using only outside resources/vendors. Incidentally, tools and standards for identifying, communicating, measuring, and mitigating bias are often housed within your Governance group, but many larger organizations have Governance deputies in each major department.
If you’re using Marketing Artificial Intelligence software/vendors, it’s essential to understand their bias standards and representativeness levels. Get this info upfront before you sign the contracts, and then have them update it every six months or so. They may squawk that nobody ever asks them this but do it anyway. (This is another one to check with counsel on first – they may have other ways they want to handle this.) Either way, it’s best to have a solid handle on what your vendors are doing because, in the end, you may be held responsible for it. This includes but is not limited to things like accuracy levels of training data, where their original data came from, how their other clients impact their models and what that impact is on your business, how often their models are updated, and so on. (You can read more about questions to ask your Marketing AI vendor here.)
Use AI to help you measure your bias. Yes, you can use AI to help identify your biases. (The delicious irony, I know.) Even better, you can use it to predict the impact of your bias(es). Should you do this? Possibly. It depends on the project. Companies have successfully used AI in the medical field, VC, recruiting, eCommerce, and more. Is it perfect? No. Does it point out different things than humans do? Yes. Is it worth exploring? Absolutely.
Survey your customers. This isn’t necessarily a must-do like some other suggestions, but it can be beneficial, nonetheless. Many companies do this by phone. I’ve also had good luck with email. The key isn’t to mention “artificial intelligence” but to understand what the customer is experiencing and what they found challenging/troublesome. Surveys can be especially helpful in eCommerce, especially around carts, internal text search, mobile experience, and hyperpersonalization. They’re also beneficial if you’re doing a lot of Voice.
Develop protocols to ensure social data doesn’t corrupt your models. This is one of those things that nobody ever tells you because they think you should already know about it. Human-generated data can create bias very quickly. If you’re allowing social data, reviews, user-generated frequently asked questions, and so on, you must ensure you are correctly accommodating for them in your models. You’ll likely want to assign importance levels to control the impact. You may need to continually adjust these levels, depending on the data’s quality and quantity and how they influence the algorithms.
Interpretation matters. Bias is a complex issue. Many companies work hard to ensure that their data is fairly represented but still stumble because they don’t know how to measure/interpret things correctly. When working on an AI project, assign someone(s) to measure and analyze the data alongside the models; have humans review the AI’s predictions and vice versa; rotate through outside vendors to do audits, etc. The more you understand the ins and outs of your model(s), the more effectively you’ll be able to manage them.
Bias is not something you can do once and then check off your list forever. You need to keep on top of it as long as you use AI. Period. Many marketers think bias is just a Legal thing, so they let them handle it. Legal should undoubtedly be involved, but bias can cause brand/social/PR issues. It can also dramatically impact your revenues. You can avoid a lot of drama by consistently analyzing and mitigating your issues. Most AI models don’t stay the same and can differ from what you start with. You must continuously review your models as they operate in the real world.
Bias is not an all-or-nothing proposition. I’ve seen companies delay dealing with their issues because they “couldn’t get it to 100%.” Guess what? Eliminating bias 100% is not likely to happen. Plus, the more your AI grows, the more risks you’ll likely have from bias. Your goal is to minimize it, especially the harmful stuff, and when you do have bias in your models, you should know how, why, and if/when you will change/eliminate it.
It’s important to remember that even if you use AI to help you figure out your bias, you will want human involvement. The Machine spots things that humans don’t, but humans also catch things that artificial intelligence won’t see. On every level, bias is a team effort.
Have a tip you’d like to share about bias? Tweet @amyafrica or write info@eightbyeight.com.
ADDITIONAL RESOURCES:
IAB Canada: Understanding Bias in AI for Marketing
Alan Turing’s Fairness, Transparency, Privacy Group
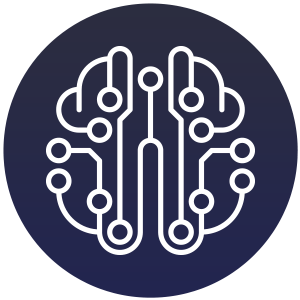
*I’m not a lawyer and I don’t even play one in the Metaverse. This article is by no means exhaustive and it’s critical that you do your own research. Full stop.