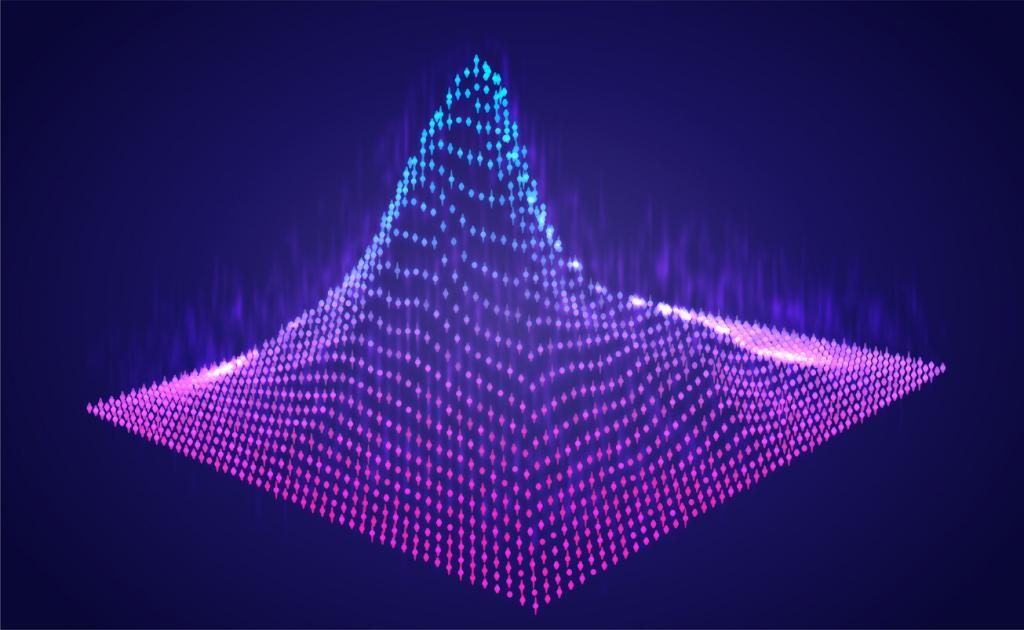
Likelihood to Purchase (LTP) predicts how likely an individual is to purchase.
For decades, marketers have determined their LTP rate. Some use old-school intent surveys or outbound telemarketing qualifiers to assess how likely someone is to buy. Others use models based on demographics, past purchasing behavior, and other variables.
Artificial Intelligence/Machine Learning has put your LTP Rate on steroids.
Instead of just a few preselected variables, AI-powered LTP models use EVERYTHING they know about the user – from traditional past purchase information and behavioral data to real-time information about the guest’s path/journey. This includes things like where the user came from (which channel); what they looked at; what they engaged with; how they navigated; what they inquired about or carted; precisely which field at which step they abandoned; what their AAUS (Average Active User Session) was; how many pages they visited; and so on.
Where do I start to calculate my LTP rate?
There’s no “one right way” to build a spectacular LTP model, and from experience, I’d recommend first building out a replica of what you already have. Run it for a month or two to ensure your model is behaving as it should. You’ll know this by comparing the model’s predictions to the data/reports/predictions you already have. Then, add one variable at a time for the first six or so variables. I know. I know. This slower-than-death approach seems like a slap in the face of the self-learning power of AI, but it’s essential that you have control over your model and, more importantly, know how/when to disrupt it. You don’t need to go slow, but if something goes awry and you can’t figure out how to fix it, you may need to start at the beginning again.
If you genuinely believe what you currently have is garbage, build something new. Just start small. Don’t try to use all the variables at once. It’s too easy to lose control. Remember, when building AI projects, it’s essential to know what/where your levers are so you can quickly disrupt your system when you need to.
When you’re confident that things are stable, feed your model. Implement ALL the things you want to add in prioritized batches so you can easily keep tabs on what’s happening. Some marketers choose to give the model access to absolutely everything instead of doing it in batches. If you do that, you must know your data bias, primary levers, and have a disruption plan at the ready.
How do I know if my model is performing?
Look at whom the model predicts will purchase and then match that information with their purchases. This process is why even antsy folks need to be patient-ish. It works best if you wait through at least one complete buying cycle. So, if your model says this person will purchase in 30 days. Look at the data in 15 days, 30 days, 45 days, 60 days, and 90 days. You’ll also want to look at 180 days and 365 days, but you only need to wait 2-3x times the average number of days to get great information. You can break all this down as you see fit, and it’s essential to include the 50% and 100% marks. They’re both excellent indicators of your model’s success.
You can also look at this daily to see how close it is. Most people find it easier to do it in clumps but do whatever’s most beneficial for you. Because people ask, I prefer it to be plotted hourly by day because I find the visualization helpful. Again, look at it in whatever way you’ll get the most actionable insights.
Be sure to take a sample of the folks the model predicts won’t purchase and look at that information. Some marketers choose to compare EVERY prediction from both buyers and non-buyers. That works too. As an aside, I often find the non-purchaser information more helpful than the purchaser information when troubleshooting our models.
Do you use LTP in your company? Have a tip you’d like to share? Have a question you’d like to ask? Tweet @amyafrica or write info@eightbyeight.com.
Read more on the Likelihoods here…
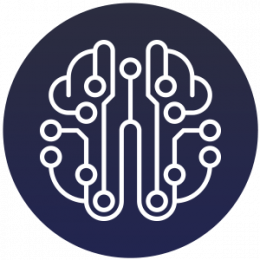