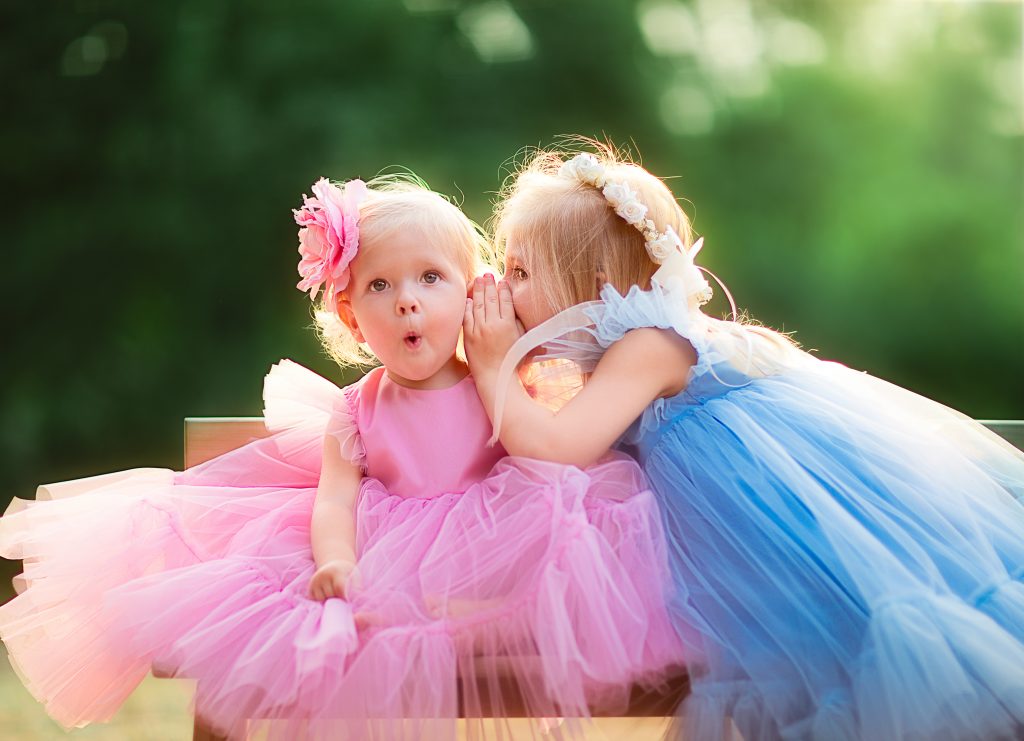
Showing your visitors items they might like and want to buy (aka Personalized Recommendations) has been an eCommerce technique for over two decades and has been used in magazines and catalogs long before that.
Back in the good ole’ days, companies made manual lists of NLPs (Next Logical Products), savings bundles, companion products, Top 10s, GBBs (Good-Better-Best lists), and the like to put on their websites and in other marketing materials.
Years later, the process became more automated yet still required some heavy lifting by your Merchandising and Marketing teams. Fast forward to today, and many companies use Personalized Recommendations completely automated and powered by Artificial Intelligence and Machine Learning. You’ll see them in cross-sells; upsells; carts and checkouts; Deal of the Days: entrance, mid, and exit pops/modals; Buy the Looks/Whole Series/Collection on Product Detail Pages; Add-Ons (often used for Accessories and low-priced items); BOGO (Buy One, Get One offers); Extended Warranty Pushes; Club/Membership Plugs; Subscribe and Save deals; and more.
When implemented correctly, Personalized Recommendations can increase your average order, decrease your DTS (Days to Sale), and improve your LTV (Lifetime Value.) Plus, they often improve customer loyalty and improve conversion rates.
What are the best places you can use Personalized Recommendations on your site?
Entry pages (including, but not limited to, your home page)
Product Detail Pages (below the main selling area, righthand sidebar, near the FAQs and reviews, etc.)
Category Pages (test first at the top and the bottom, then try top right, then left)
Drop/pop carts/modals
View Cart Page
Checkout Pages
CheckPops (pops/modals within the checkout – typically used right before the user finalizes their order)
Thank you pages (email sign-up, catalog sign-up, order confirmation, My Account, order tracking, and such)
Confirmation pops/modals (often overlay on confirmation-type pages)
Live chat modals and pops (position is critical)
Why is AI/ML a game-changer in Personalized Recommendations?
Real-time fluidity.
Personalized Recommendations powered by Artificial Intelligence/Machine Learning typically work better because the systems can process, analyze, and learn from so much more data. This includes the historical data that most AI/ML packages are initially powered by and current/ongoing on-site activity. The more history you have and the more you know about the product and/or that user (person) today (in the present), the better your recommendations are likely to be.
Current/ongoing site activity can make a HUGE difference. Trends and preferences change. Products improve. Brands evolve. People get older and want/need/think about different things.
What kinds of data should you look at besides historical data?
Here are some of the things that can make the most difference:
New visitor or repeat visitor?
Buyer or prospect?
AAUS (Average Active User Session) tells you how much time they’ve actively spent on your site. (Time spent/# of pages viewed – this is different from regular Active User Session)
Device information indicates whether they’re shopping/browsing from their desktop computer, a mobile device, a tablet, or something else (car, phone, refrigerator, TV, etc.)
Channel Information tells you where they came from. Direct? Email? Old-school PPC? Shopping? SMS? Organic? Social? Etc.
Search Query Information looks at internal text search and, more specifically, what keyword(s) the user typed in
View/Browsing History shows you how many and what pages/products have been viewed. (Using this correctly can have one of the BIGGEST impacts on your results.)
Adoption information looks at whether users added something to their cart and where/how they did it. It also looks at cart starts, a critical but often ignored metric. If the user didn’t adopt, what meaningful action(s) did they take? Did they sign up for your emails? Request a quote? Start the personalization/customization process? Choose a size but abandoned on the color? Etc.
Where are they located? What’s their proximity to your product? (Proximity looks at how close the user is to receiving your product – in other words, how fast will they get it?)
Are they coming in with a coupon or a promo code? What’s the offer?
Product type? Especially beneficial if the product is frequently ordered (aka consumable) product.
Brand sentiment?
We’re doing an excellent job on our own with personalized recommendations. Will AI do a lot better?
Usually but not always.
The challenge with using AI/ML is that it is not as “plug-it-in-and-it-prints-money” as vendors sell it. So, if you have a solid system on your own, just replacing it with whatever “AI solution” someone sells, can be a colossal mistake. (And frankly challenging to recover from.)
If you’re willing to put in the effort to set it up, train it and then maintain it CONSISTENTLY, it will likely outperform your manual processes. And typically by A LOT. If you’re not willing to commit to doing those things, keep your current system and use your money elsewhere. Personalized Recommendations can run awry and negatively impact things quickly.
How can I make our Personalized Recommendations system the best it can be?
Account for holidays/seasonality. If you’re like most businesses, there is a time (or times) of the year when you’re way busier than others. (For example, Christmas for retailers or the government fiscal year for B2B companies.) If you’re selling different items during that time, you need to account – and possibly adjust – for that. Otherwise, you’ll be recommending Christmas Wrapping Paper at Easter.
Figure out the best way to put New Items in the rotation. (If Exclusive, Clearance, Sale, or something else is essential to you, then you’ll want to account for them/those too.) Most AI/ML relies on past data, so if there’s no past data (New Items) or the past data isn’t great (Overstock, Clearance), you may want to make special accommodations. You’ll want a process for introducing new things to the recommendations AND a plan for getting the old/no longer available/etc. out of the recommendations.
Please pay attention to your AOV (Average Order Value) vis-a-vis the item(s) you’re recommending and where you are recommending them. Companies often implement “global” Personalized Recommendations that are the same from the home page to the cart/checkout. If you’re upselling and cross-selling on a PDP (Product Detail Page), a Dropcart, and a Checkout Pop/Modal, chances are specific products will perform better than those you’re dynamically featuring on the home page.
Test. Test. Test. Marketers tend to over-test piddly things on a website (like button colors) yet do very little (or no) testing on the BIG SWING things that move the needle. For example, test whether your dynamic recommendations should be based on the items themselves (you like this bracelet, here’s another bracelet that you will like), the user/market (you like cats, so you’ll probably like candles), or a combination of both. Frequently Bought Together, Bundles, and so on have their place, and there are other solutions for different areas on your site that you should test. Incidentally, it’s essential to test each area where you use Personalized Recommendations individually and as units or small groups.
Sensitivity to reviews. Reviews are like grocery store coupons: people use/read them or don’t. If a user is influenced/influenceable by reviews, then showcasing well-reviewed (# of stars and/or # of reviews) first can make a BIG difference. Mileage varies on this, so it’s vital that you test it. You will find a formula that’s right for you.
Measure more than just conversion. So many marketers are OBSESSED with conversion, yet they forget that your conversion is a function of traffic. Want to double your conversion overnight? Block/isolate your bad/weak traffic. Your conversion rate will increase, but your overall revenues may remain the same/decrease. But I digress…. Measure overall conversion as you will but also remember…. One of the most important things to track in Personalized Recommendation Programs is adoption to cart. (Out of x viewed, how many people put the item in their basket? How many times was the item shown, and how many times was it added to the basket? (If you have a fussy product with sizes, colors, personalization, etc., it’s worth it for you to measure cart starts. Cart starts measures attempts and carted items.) On another note, it’s helpful to have an overall revenue/pieces/AOV # (how much you’ve increased/decreased things) for the entire experience and each area.
Consider utilizing 3rd party data overlays. This recommendation isn’t for the faint of heart, but it’s worth exploring, especially if you feel you’ve tapped out. It’s not hard and relatively inexpensive, but you need to be strategic about the process. Otherwise, it won’t amount to anything. Use a reputable provider, and please don’t use all the new variables simultaneously. Instead, prioritize the list and implement/test them accordingly.
Have a question about using AI to personalize your recommendations? Have a tip you’d like to share? Tweet @amyafrica or write info@eightbyeight.com.
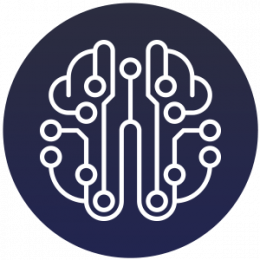