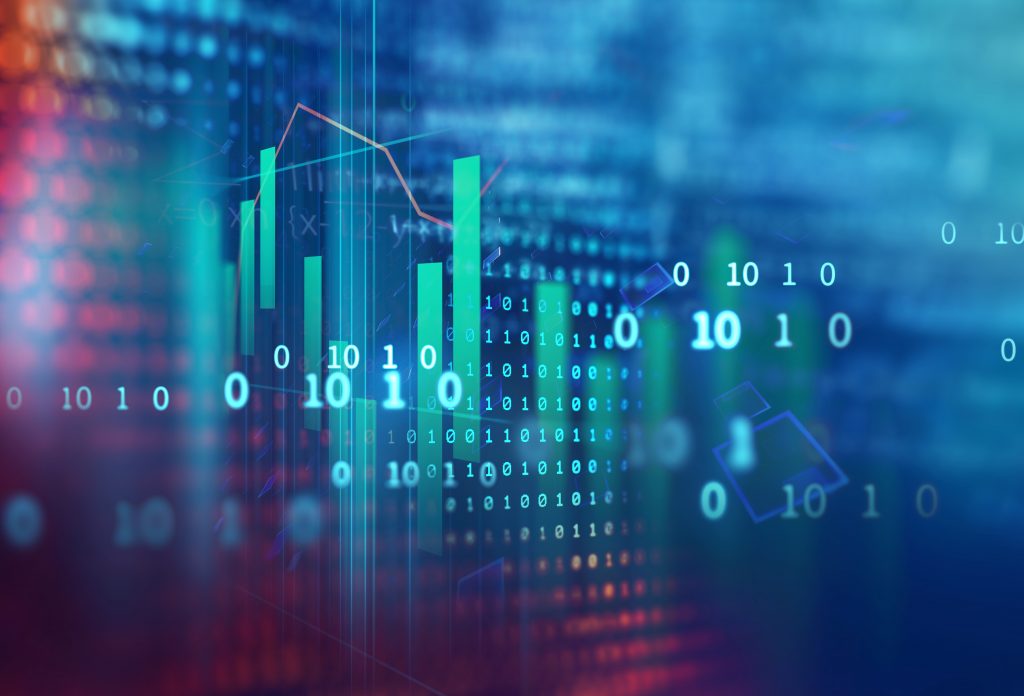
You’ll hear it over and over again…
“Data is the new oil.”
“Data is the new gold.” (Technically, it’d probably be Rhodium, but who am I to quibble?)
“There is no Artificial Intelligence (AI) without data.”
But what does that mean when it comes to your Marketing projects using Artificial Intelligence and Machine Learning (AI/ML)?
Quite simply, it means that your data will be THE key to your success. Full stop.
Most AI/ML projects don’t exist unless humans set them up first.
The systems do what we tell them to do.
We give the goal.
We assign the purpose.
The systems figure out how to reach the objective(s) through lots of trial and error.
No matter how chi-chi-la-la the system is, it won’t work without data.
Data doesn’t do anything on its own.
If your data is bad, the outcome will likely be bad/poor.
If your data is good, the outcome will likely be good/beneficial.
“Good” data doesn’t need to be perfect. It must be clean, relevant, organized, properly labeled, and complete. Incomplete data tends to be difficult for systems to analyze. It’s not impossible. Just messy and often unreliable.
These days, most Marketing AI projects are designed to predict future outcomes. To do that, they use past (aka historical) and current (aka real-time) data.
The better the data, the better the outcomes.
IN THE WORLD OF MARKETING AI, THERE ARE THREE MAIN KINDS OF DATA
Bullseye Data (aka Source Data, First-Party, and/or First-Tier Data)
This is the data that you’ve collected. You own it. It’s yours.
This is typically the most important type of data in your arsenal. As long as you can segment it, the more history you have, the better.
It’s important to note that many marketers think Bullseye Data is just buyer/purchaser data. It’s not. Bullseye Data includes online and offline buyers AND inquiries. Inquiries could be people who sign up for a free newsletter or to receive text messages, podcast and video subscribers; webinar watchers; whitepaper downloaders; live chatters; etc. – anyone who has shown an interest in your products/services.
Bullseye Data also includes intent data: abandoned cart and/or lead form information; cookie information; time spent on site and page measurements; the number of pages looked at and specific browsing stats; app usage; viewing (video) stats; and so on. Anything that indicates the user’s propensity to purchase/interact with your business is Bullseye Data.
When you’re shooting at a target, you aim straight for the bullseye in the middle. It’s the #1 thing you want to hit. When we talk about data, your Bullseye Data is THE biggest thing you want to get right.
2nd Party Data (aka Second-Tier Data)
This data is someone else’s first-party data. They’ve collected it. They own it. They’ve allowed you to use it. Before Co-Ops, the direct marketing world was built on this kind of data. Companies, even competitors, frequently exchanged buyer lists with each other.
3rd Party Data (aka Third-Tier Data, Compiled Data, Marketplace Data, Aggregated Data)
Third-party data is aggregated data collected through a vendor, co-op, profiler, compiler, or marketplace. There are often overlays (demographics, infographics, neurographics, psychographics, etc.), but in many industries, it’s just combined data.
Depending on the industry, the aggregator may not have a direct relationship with anyone on the list. When working with an aggregator, it’s important to know how they got their data and what/how they’re following privacy and security laws and standards. Be sure to ask how they identify and parse fraudulent data as well. (More on this below.)
Third-party data often gets a bad rap. It can be INCREDIBLY useful to you in your online and offline marketing efforts if you understand how it has been compiled and how current it is. (The freshness of the data often becomes more important when it’s not yours.) Just make sure you do your due diligence. There are big-name vendors doing smarmy things these days, and you don’t want to get caught up in any of the drama, I can assure you.
“What type of data is best?”
It depends on what kinds of models and systems you are building. Many AI consultants like to push their clients to use 1st party data with 3rd party overlays. This is not necessarily bad as long as you know why you need the 3rd party data. Are you using it for scale? To fill in gaps? To make better predictions? To get the information you don’t have (competitive information, for example) and want? Or do you need it because your data is such a dumpster fire that it’d be easier just to buy someone else’s? If the latter is indeed the reason, you should go back to the drawing board and clean up your Bullseye (1st Party) Data before you start mixing it with anything else.
As an aside, in the AI world, third-party data is often handled differently than old-school catalogers, and direct marketers are used to. If you’re a legacy company and ruled out third-party data years ago because of how smarmy the industry was back then, I encourage you to look at it again with a fresh perspective. I’ve done a lot of AI projects where most of the success was due to the addition of outside data. You don’t need it for everything, but it can be a BIG difference maker for some stuff. Additional data can be incredibly beneficial for developing new markets, finding new audiences for new/existing products, and other things where making solid predictions with solely 1st and/or 2nd party data may be challenging.
“What is Zero-party data, and why isn’t it included above?”
Zero-party data is data that your visitors intentionally share with you. It includes things like their communication preferences (from your email preferences center, for example); survey information that the visitor has proactively shared; personal information that they’ve specifically offered you when signing up for offers, whitepapers, and such; live chat information that they’ve consented to you using for marketing purposes; and so on.
It’s not included above because, for many companies, it’s difficult to segregate. For others, it makes things overly complicated. (This is especially true for companies with sales organizations.) Zero-party data is more transparent. It can build trust. And if it’s easy for you to capture and segment, by all means, do so. However, please don’t let it paralyze you if you cannot collect it now. (Please note: different countries have different rules for this. Consult with your lawyer for specific recommendations.)
“Where does all the third-party data come from?”
Way back when, it came from things like surveys, warranty cards, loyalty card sign-up, subscriptions, property sales, census information, directories, registrations, telephone books (yikes!), etc. Now it’s much more complex, tying in all sorts of online activity data from apps, websites, social, ads, search, email, cookies, etc., and real-world data from location information, store conversations/browsing, etc.
Most of the good third-party data now come from just a few main suppliers. If on the off chance, you’re using a secondary or tertiary source, they still may be using one of the main suppliers to hygiene and/or enhance their files.
“Does my data really all need to be in one place?”
For your best chances of success, you need rich and accurate data. The higher the quality of the data, the better the outputs. The highest quality marketing data usually come from companies that have prioritized centralizing it.
Many companies have data that they haven’t compiled internally yet. For example, many businesses don’t log all their incoming Customer Service data. This includes chats, customer service, and sales emails, support queries and downloads, and so on. Some companies don’t log all their activity data. Others house their sales data and/or their Voice data in entirely different systems. I could go on, but you get the drill. Wherever your data is, it’s best to get it ALL in one place and then put a hierarchy together that ranks it from most to least important.
After that, you can figure out where you have missing gaps. Do you need more demographic overlay information? More behavioral data? Something else? Does your buyer information have recency, frequency, monetary information, and affinity groups? Knowing what type(s) of info you need to supplement will help you choose the right data vendor(s) for your needs. The amount of information offered these days can be incredibly overwhelming, so it’s best to identify what you need before you start looking.
“One of our vendors recommended that we enrich our data because it’s not good enough. How do I know if this is true?”
This question comes up a lot. The answer typically depends on what type of project you’re doing. Data enrichment combines your first-party (Bullseye) data with other data sources to give you a better picture of your audience. It’s excellent at fleshing out customer and prospect info/profiles. It’s fantastic at finding “like” audiences (similar customers to the ones you already have.) From a Marketing AI perspective, enriching your data can be highly beneficial as more robust datasets often garner better predictions. (Please don’t underestimate this.)
At the minimum, your data should be:
Accurate. Relevant. Valid. Consistent.
Labeled appropriately.
Clean (recently and frequently been hygiened.)
Comprehensive for the project. To train your models properly, try to have all the data upfront. I know many vendors say you can “just add things later,” and although this is true, it’s often not advisable. In a perfect world, all your fields will be filled in, and you’ll have all the necessary information to do the job before you start training your models.
Worthy of being included in the project. As I’ve mentioned, I’m the person who wants ALL the data, and it’s important to curate what you use. If something is not required for you to do the job and you wouldn’t bet your house on it, don’t use it to train your model. Add it as a test later on and see if it’s viable for permanent inclusion. Remember, whatever elements you use at the beginning may be maximized (scaled) in the future.
And, about your vendor… There are several smarmy vendors in the data enrichment space. They’re uber-aggressive about telling folks that their data sucks without ever having looked at the data. If a salesperson tells you you need to use their product to improve your data, ask them for specific examples of how they could help and accompanying audience counts and costs. The good ones will do this for FREE. The others will slink off to their next victim.
“Is there such a thing as too much data? Seems like the more, the better.”
More data is better as long as it’s relevant.
If your data isn’t clean and/or isn’t prioritized, more won’t necessarily help you and can hurt you, depending on how/where you use it. (Think Garbage In, Garbage Out.)
In the old-school world (catalog, traditional direct marketing, 2-step, etc.), throwing everything at the wall and hoping something stuck was easy. With AI/ML projects, that method is often the fast track to disaster. High-quality data allows you to build better models, saves time (less rabbit chasing), and makes it faster to identify bias and maintain compliance.
In the interest of full disclosure, I’m one of those people who wants ALL the data. Every last speck of it. Once we get it, we prioritize it, review it, and then test/train. I don’t use even a drop of the data until I am confident it’s suitable for whatever AI-enabled solution we’re using. If you don’t do this and just dump everything into your AI haphazardly, the system is forced to sort it out independently. Once in a blue moon, this works, but more than not, it’s a raging hot mess. You end up having to disrupt too early or start over. Plus, there are often negative long-term ramifications. It’s worth the extra time to sort things out upfront.
Can I still do AI projects if I don’t have a lot of customer data?
First, it’s important to remember that not all AI projects need customer data. Without it, you can do lots of things (for example, content generation).
Second, there are many times when you may need to work with small datasets. If you do, make sure you need to use AI for the project. (A spreadsheet may do just fine.) If you decide to proceed with the project, make sure that the little data that you do have is rock solid.
One of the biggest fallacies of AI is that you need to have tons of data to make AI work. For the record, this is just not true. You can build good AI with a modest amount of data. The issue is that the upfront time/cost to do this may outweigh the short-term benefits. The good news is that more and more cookie-cutter(ish) models are becoming available, and they’re often free or low-cost. Interested in learning more? Start at AWS, Google, and Microsoft.
We’d like to try using a third-party data vendor to enhance our customer file. I’ve researched several, and they all seem the same. Should I pick the one who gives me the best price?
I agree. At first glance, they all look the same(ish). When you dig deep, though, you’re likely to spot vital differences in their ethics and their data collection processes. As an aside, the team/rep you’ll work with on an ongoing basis is also critical. This is where you may cut many vendors from your list of possibilities – you’ll want someone who understands your needs and is trustworthy. But I digress… When embarking on data enrichment or hygiene projects, I recommend you ask the potential vendor the following:
Where does your data come from? What kind of consent have you gotten to obtain this information? How recent is the information? (Data freshness is something you’ll want to ask many questions about. Some of the bargain-priced vendors have older-than-dirt data in very snazzy packaging.)
How do you keep your data clean? How do you cull out bad, invalid, and fraudulent data?
What kind of information can you provide me? Demographic data? Behavioral data? Activity information? Do you have anything unique that your competitors don’t? How current is the information? What’s your hygiene process? How is your data formatted?
How much data do you have that’s relevant to me? This sounds like a throwaway question, but it’s important because every data vendor and their pet pig seems to say they have billions of records and selects. Then, when you start digging into it, they don’t have the particular data that you need to give you the best insights. (This happens the most in B2B, but it also happens a lot in niche B2C.)
Do you use a scoring system for your data? If so, how does it work? If you plan to use the scored data from your vendor in your projects, it’s essential to dig into how it’s been scored. (Vendors often used AI/ML in their scoring systems. This can heavily influence your models, especially if you’re using them in your testing and training.)
Tell me about your privacy and security compliance. Are you compliant with GDPR? CCPA? COPPA? Do you have a data transparency statement?
Do you have a data satisfaction guarantee?
What is text mining, and should I include it?
Text mining (aka data mining) extracts insights from text. Marketers often use text mining in projects that involve reviews, live chat, customer service call logs, inbound emails, SMS, etc., as it turns unstructured data (videos, images, audio, comments, reports) into structured data.
Text mining can be incredibly helpful if you are doing projects where you want to integrate user sentiment into your models. It’s a quick way to get a lot of data into a usable format.
What is data governance, and do I need it?
Data governance means establishing internal standards about how your data is gathered, stored, processed, disposed of, and accessed. Data governance ensures that your data is accurate, complete, and doesn’t get misused.
More about Data Governance can be found here.
What’s the biggest thing I should know about Marketing Artificial Intelligence and data?
Garbage In Garbage Out can be forever when it comes to AI. AI is a hot topic right now, and many companies are rushing to “do AI” as if it’s as easy as obtaining cocaine in Bolivia. (Newsflash: it’s not.) They dump all their information willy-nilly into a machine-learning model and let it run wild. Sadly, that’s precisely what it does – resulting in missed goals, inaccurate predictions, bad outcomes, and a host of other negatives. You must train your models properly.
Training data is critical. It impacts the quality of the insights you’ll get more than any other element, including your modeling techniquess.
One of the best things about AI is that it processes and analyzes oodles and oodles of data in real time and at scale. This is all done at a blazing speed that humans simply can’t replicate. When properly managed, your Marketing AI models will get better over time. As the AI gets more robust, it will make better predictions. As the models get older (the longer they have the data), they typically get more precise, making better predictions, and giving you better insights and actionable information. (Reason #175918501 you’ll want to start using Marketing AI sooner rather than later.)
Have a question about marketing data? Have you like to share a tip about using Artificial Intelligence Data in your business? Questions you’d like to ask? Tweet @amyafrica or write info@eightbyeight.com.
A Down-and-Dirty Definition. (Read more about these here.)
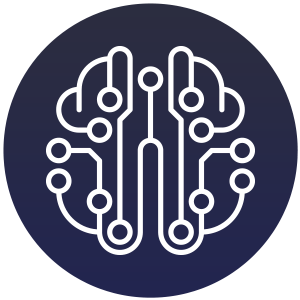